The Accuracy Gap: Why generic AI falls short in energy management
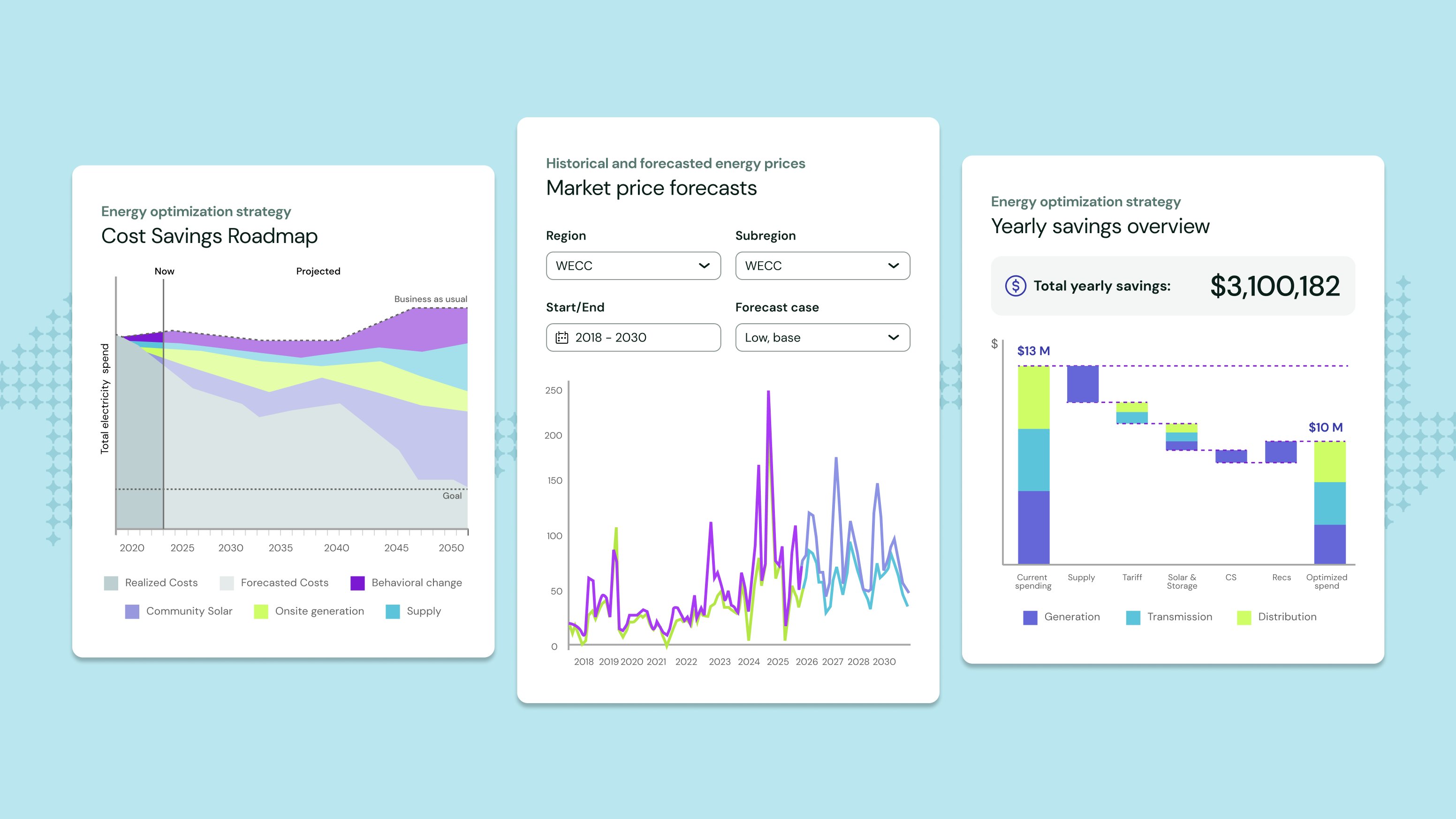
Artificial intelligence has rapidly evolved from a futuristic concept to a competitive necessity. In today's business landscape, organizations across industries must leverage AI or risk falling behind more agile competitors. But the reality is that today's AI tools — despite their impressive capabilities — are insufficient to deliver transformative business outcomes on their own.
While AI offers powerful automation and decision-making capabilities, it needs human oversight to ensure accuracy, responsible implementation, and meaningful application. The technology must be guided by industry expertise and fed with high-quality, relevant data to produce actionable insights that drive real value.
In this Q&A with Udit Garg, Arcadia’s VP of Product & Engineering, we explore why generic AI solutions fall short in today's complex energy landscape — and how Arcadia's experience informs our strategic approach to leveraging AI.
Why did Arcadia start integrating AI tools into its products?
AI integration at Arcadia has been an evolutionary journey rather than a sudden shift. When I joined a few years ago, the foundation was already in place — we had developed sophisticated AI features like storage and EV charging optimization models, and were leveraging AI throughout our data pipeline to transform unstructured data on scanned bills into actionable information.
Still, in one of my earliest conversations with Kiran (our CEO), he emphasized wanting to do a lot more with AI, recognizing the immense opportunity to dramatically increase efficiency and deliver greater cost savings for our customers. That vision has become a central pillar of our product strategy, guiding our team's focus and innovation efforts over the past several years.
How does Arcadia’s deep experience in the energy data space differentiate you from companies that are coming to market with AI-first solutions?
Our years of experience in energy data has given us a crucial understanding that many AI-first products lack: the absolute necessity of accuracy in this industry. The 5-9% accuracy gap between typical AI solutions (90-95%) and our standards (98-99%) becomes critical when managing energy infrastructure and making multi-million dollar investment decisions.
This understanding drives our hybrid approach. Rather than pursuing AI for its own sake, we strategically integrate it with our established data infrastructure. This combination allows us to maintain the reliability our customers demand while enhancing our capabilities.
Additionally, the energy domain modeling is crucial to our platform and customers. Over time, we've developed a robust, battle-tested, and universally applicable energy data model that works across 10,000+ utilities. This level of standardization is something no AI model or startup can replicate or even approach. Our customers depend on this standardized model to run their businesses efficiently, without having to account for the extreme heterogeneity across utilities — a complexity that would otherwise lead to "garbage in, garbage out" scenarios with their data.
Overall, we've built our solutions with a deep appreciation for the complexities of energy data across thousands of utilities and regulatory environments — essential context that AI-first companies often underestimate when entering this space. This domain expertise, accumulated over years of solving real-world energy challenges, enables us to deliver AI-enhanced solutions that meet the exacting standards our industry requires.
How does Arcadia determine when to use in-house tools versus third-party LLMs?
We make our decisions based on several key factors.
- For traditional machine learning applications — prediction and classification models — we've determined that building in-house makes the most sense. The tools for developing these data-driven models are well-established, and this approach gives us maximum control over the parameters and accuracy for our specific use cases.
- For generative AI, our decision-making balances cost efficiency, data security, and time-to-market considerations. Most critically, we prioritize absolute protection of customer data. Currently, this analysis has led us to partner with third-party providers that ensure customer information is never used to train outside models. This non-negotiable requirement protects our customers' sensitive energy data while still allowing us to leverage advanced AI capabilities.
While we anticipate eventually reaching a scale where the economics favor hosting our own LLMs, our current evaluation shows substantial value in third-party tools — provided they meet our strict data protection standards that ensure customer information remains fully secure and private.
The AI Accuracy Gap: Why Energy Management Demands More
As our discussion with Udit reveals, the limitations of general-purpose AI are particularly significant in energy management. The gap between what standard AI tools can deliver (90-95% accuracy) and what effective energy management decisions require (98-99% accuracy) may seem small, but represents the difference between reliable insights and potentially costly errors.
This understanding guides Arcadia's approach to AI integration, informing critical decisions about which technologies to develop in-house and which third-party solutions to adopt — always with an eye toward maintaining data security and meeting the exacting standards our customers demand.
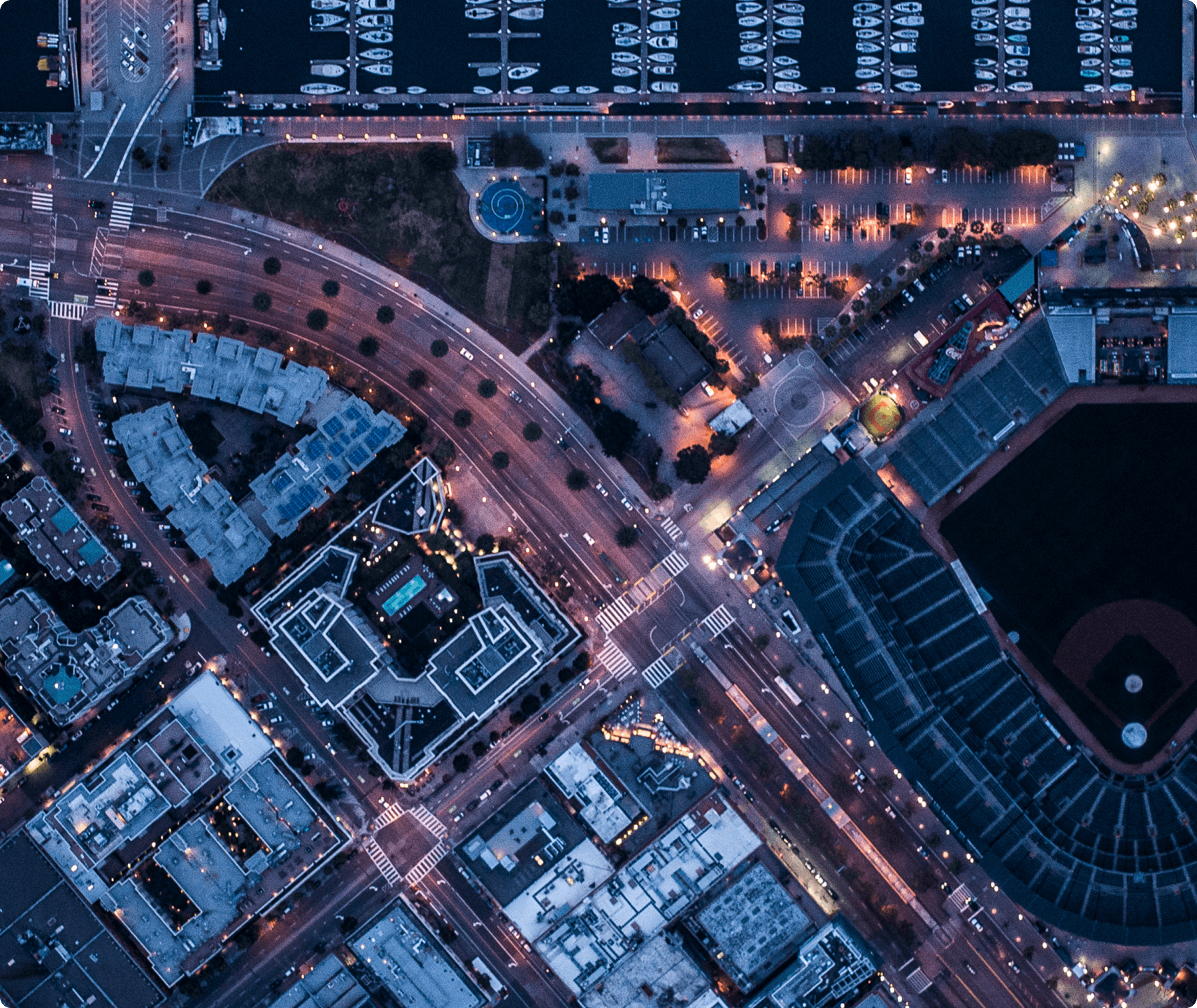
Leverage Arcadia's data, technology, and expertise to unlock energy solutions for your business.
Contact us